In Formula One racing, the margin between victory and defeat can be measured in milliseconds. Teams spend countless hours fine-tuning their cars—adjusting aerodynamics, engine mapping, suspension, and tire pressure—because even a minor tweak can shave precious time off a lap. This relentless pursuit of optimization requires the blending of hard data with human intuition.
The world of clinical AI may seem far removed from the racetrack, but it operates on a similar principle: success depends on meticulous tuning. In this context, the AI model is the engine, data is the high-octane fuel, and the pit crew is a team of clinicians and data scientists working in harmony to achieve peak performance. The perfection of clinical AI tuning lies in balancing technical precision with clinical insight so that AI tools not only run fast but also run true on the complex track of healthcare.
At Clinithink, we approach clinical AI like a championship racing team—recognizing that each healthcare environment represents a unique "track" with its own challenges. Medical terminology varies by institution, clinicians develop their own shorthand, and facilities adopt unique "house styles" in documentation. To best adapt to the unique characteristics of each “track”, we tune our Engine Encoding and Abstraction capabilities to make sure they capture the clinical findings accurately (Encoding) and focus them with laser precision on the relevant concepts for the desired use case (Abstraction).
Why Tuning Matters in Clinical AI
While foundational clinical data remains broadly consistent, every healthcare setting has its own linguistic fingerprint. This can manifest as:
- Institution-specific abbreviations and acronyms
- Staff and department naming conventions
- Local variations in describing conditions and procedures
- Documentation templates with embedded guidance text
Without proper tuning, these variations can lead to misinterpretations or missed insights in AI systems, whether based on LLMs, classifiers, or CNLP. The tuning process for CNLP is similar to that of fine-tuning Large Language Models, where the system parameters are adjusted based on how far results deviate from expected outcomes.
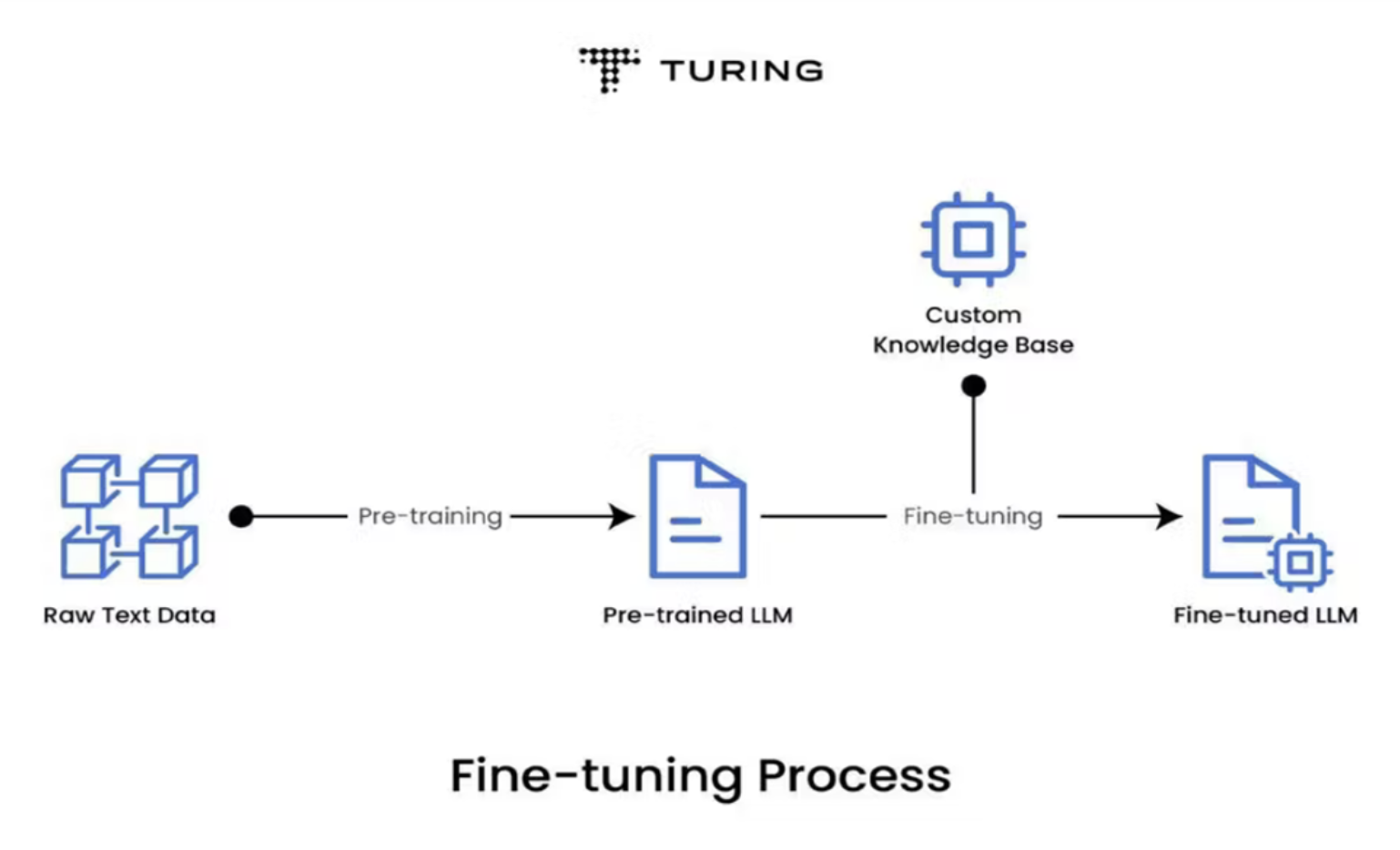
The Clinical Tuning Challenge
Consider a few real-world examples that demonstrate why tuning is essential:
- A nurse named "Lye" being incorrectly flagged as a clinical term
- A cancer institute's name being misinterpreted as a clinical finding
- The UK postal code for Peterborough ("PE") being encoded as "Pulmonary Embolism"
- Standardized patient educational text being treated as clinical observations
Much like Formula 1 engineers calibrating a car for a specific race circuit using telemetry data, our clinical AI requires precise adjustments for each new environment it encounters, in order to maximize the accuracy of the CNLP Engine and Abstraction capabilities
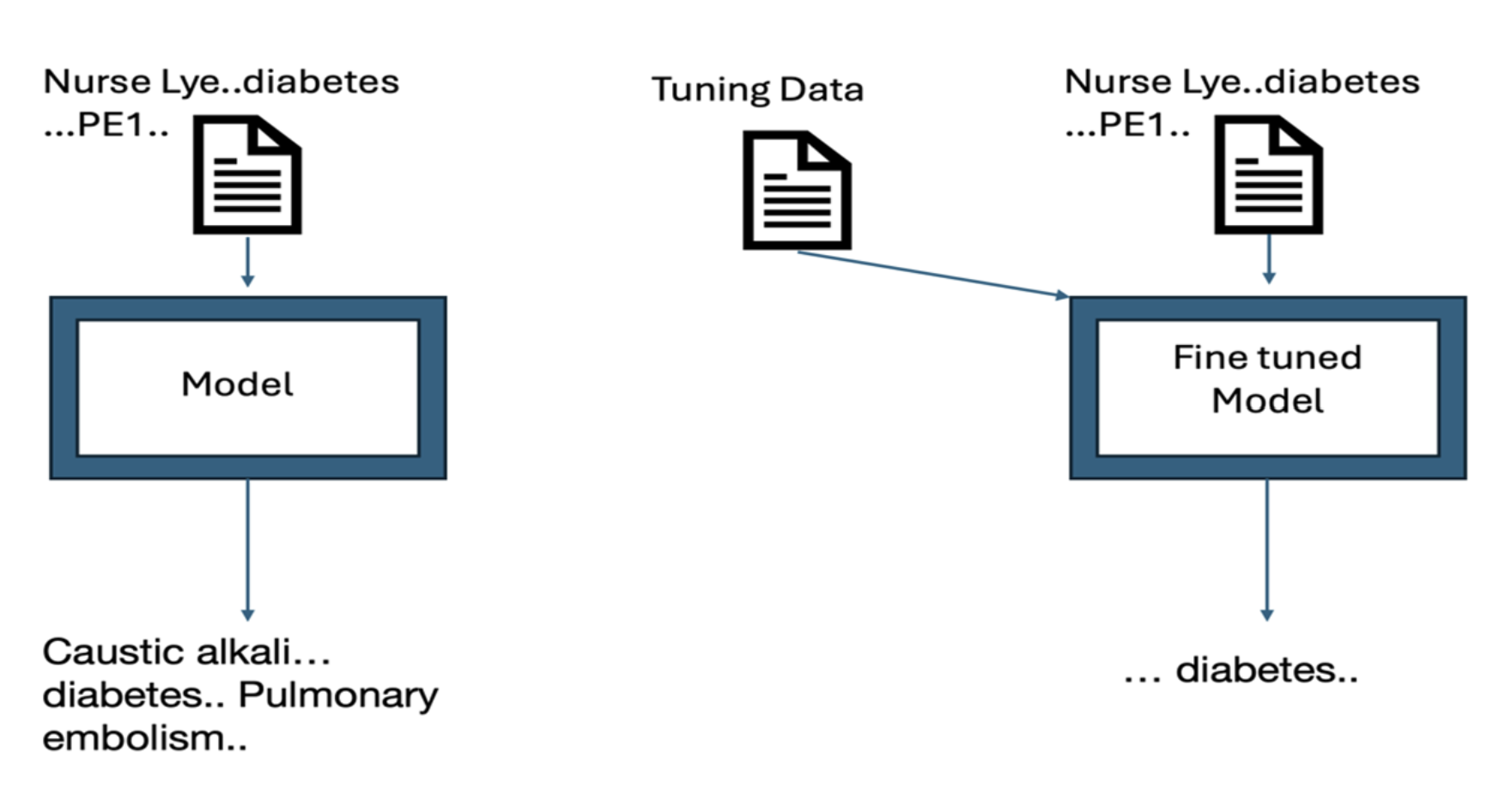
- Document Structure Recognition
Identifying new section headings specific to an institution (e.g., "reason for visit," "clinical impression") - Terminology Adaptation
- New acronyms and abbreviations
- Locally-used synonyms for standard terms
- Unknown words requiring classification
- Contextual Understanding
- "High-value noun phrases" — frequently occurring word combinations with clinical significance
- Distinguishing between actual clinical findings and non-clinical content
- Nuisance Text Filtering
Identifying and properly handling standard text that appears clinical but serves other purposes (e.g., insurance disclaimers, patient instructions) - Highlight problematic acronyms, headings, or encoded terms
- Override resource settings to stop inappropriate expansions
- Add new specialized term definitions
- Test changes in real-time using natural language functionality
- Save customized configurations for future processing
- Want to see it in action? Contact us to learn how Clinithink can transform your clinical data strategy.
- Curious about the nuts and bolts of Clinical NLP? Check out our blog post on Advanced encoding and querying in AI.
- Looking for a deeper dive? Download our latest white paper, “Not All Healthcare AI is Created Equal,” for an in-depth look at how ontology-driven approaches outperform purely predictive models in today’s complex clinical landscape.
The Tuning Process
Similar to LLM tuning process for language and context, the CNLP tuning process focuses on several key areas relevant to CNLP, to maximize accuracy
For nuisance text specifically, we employ a neural embedding model (based on Meta's StarSpace) that learns from each client implementation, continuously improving its ability to distinguish between clinically relevant and administrative content.
Interactive Calibration: Empowering Users
To come back to the metaphor of a race car, success in Formula 1 depends on seamless collaboration between drivers and engineers. We've brought this same collaborative approach to clinical AI tuning. Our systems allow clinical users to:
This interactive capability dramatically reduces the time required to optimize the system for a specific clinical environment. Changes can be implemented immediately, with the platform learning from each adjustment.
The Clinithink Difference
What sets our approach apart is the balance between standardization and customization. While we maintain a robust foundation of clinical knowledge, our tuning process ensures that each implementation adapts to its unique environment without compromising accuracy.
The result? A clinical AI system that speaks the same language as researchers and clinicians—understanding abbreviations and shorthand, recognizing documentation patterns, and utilizing contextual information.
The outcome is a solution that is customizable to create results tailored to perform RWE and population health analysis in Life Sciences, by virtue of the configurable and tunable CLiX Engine Encoding and Abstraction capabilities. This capability ensures that CLiX delivers insights that reflect true clinical reality.
By fine-tuning our models to each scenario’s unique characteristics, we ensure that the valuable clinical data contained within notes and records is accurately captured and interpreted, regardless of how it's expressed.
Ready to learn more?
At Clinithink, we believe healthcare AI should be both fast and verifiable. We’ve designed our CLiX engine to deliver robust clinical data extraction and coding at scale, powered by SNOMED CT and enhanced by a dynamic attention mechanism built for the real-world demands of medicine.
By tuning AI for specific clinical use, we offer a proven way to get ahead in clinical research—and we believe that’s exactly what healthcare needs most right now.